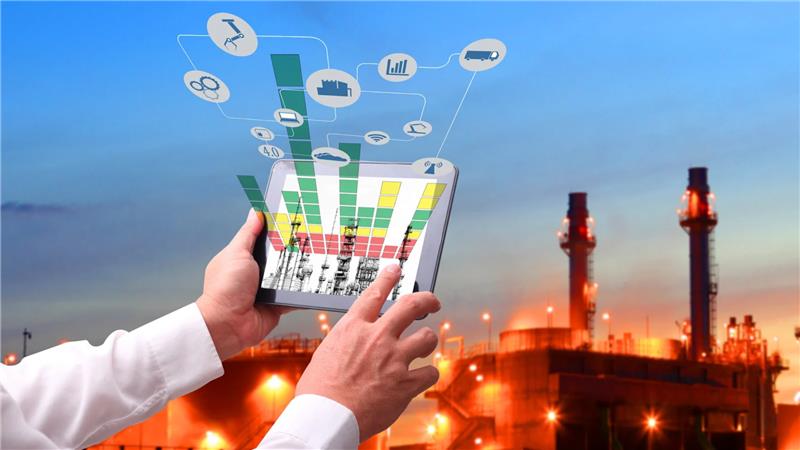
Key Takeaways
- AI-driven digital twins enable manufacturers to monitor, analyze, and optimize smart factories in real time, ensuring improved efficiency and reduced operational disruptions.
- Predictive AI-powered maintenance can identify early failure signs, allowing manufacturers to prevent costly downtimes and extend equipment lifespan.
- Process optimization through AI and machine learning helps reduce resource wastage, eliminate bottlenecks, and enhance production workflows for greater profitability.
- AI-powered simulations provide manufacturers with predictive insights, allowing proactive decision-making without interrupting factory operations.
- Seamless data connectivity between IoT sensors, AI analytics, and enterprise systems ensures that digital twins dynamically reflect real-world conditions.
Artificial intelligence has helped numerous industries thrive, and manufacturing is one of them. Because AI and industry technologies are advancing with time, it has opened doors to innovative manufacturing, one where digital twins have all the attention.
A digital twin is a virtual replica of a system, procedure, or object. It updates itself in dynamic environments using advanced AI models, suitable techniques, and real-time data. The integration of digital twins with artificial intelligence is redefining the manufacturing industry. The manufacturers can focus on priority tasks like maintenance strategies. However, one of the best benefits of digital twins is that they take pride in offering a detailed view of factory workflow. This means that the manufacturers can prepare themselves beforehand to avoid unwanted situations. Apart from this, they can find out if there are any bottlenecks. This way, the manufacturing process is improved, and the companies can proliferate.
AI-driven digital twins are indispensable, enhancing quality control and production planning and allowing manufacturers to make independent decisions. Now that manufacturers are familiar with advanced technologies, they have identified how to implement them. Therefore, the future of manufacturing is in good hands
Also read: Why Is Cloud-Agnostic DevOps Key to Cross-Platform Growth?
Understanding Digital Twins in Smart Factories
In the era of Industry 4.0, digital twins have emerged as a game-changing innovation, redefining manufacturing processes and operations. A digital twin is a virtual model of a physical asset, process, or entire system that mirrors its real-world counterpart in real time. These intelligent replicas continuously receive data from IoT (Internet of Things) sensors, enterprise systems, and advanced analytics platforms, allowing manufacturers to monitor, analyze, and optimize operations dynamically.
Digital twins create a comprehensive and constantly updated representation of factory assets by combining real-time data streams with AI-powered analytics, simulation, and machine learning models. This allows manufacturers to gain valuable insights into equipment performance, production workflows, and supply chain dynamics. With the help of predictive analytics, digital twins can monitor potential failures and suggest suitable actions before any challenge arises.
How Do Digital Twins Work in Smart Factories?
Are you curious about how AI-driven digital twins work in smart factories? We have listed the steps below:
Data Collection
Any sensor or IoT device installed on production lines and machines can gather data. All this data concerns energy consumption, pressure, temperature, vibration, and more. Once the data is collected, it is transmitted to the digital twin for further analysis.
Data Integration & Processing
All the collected data is ready for integration with enterprise systems, including PLM (product lifecycle management), ERP ( enterprise resource planning), and MES ( manufacturing execution systems), to create a suitable view of workflow.
Simulation & Analysis
Machine learning models and AI-driven algorithms examine data, consider various operational situations, and determine the impact of several manufacturer decisions on asset longevity.
Decision Support & Automation
Any insight produced by a digital twin allows manufacturers to enhance process efficiency. It also helps automate decision-making with little human intervention.
Key Benefits of Digital Twins in Smart Factories
Now that we know how digital twins work, let us shed some light on their advantages:
Real-time Monitoring
Digital twins offer a live view of factory workflow. This helps manufacturers track how the machines are functioning without visiting the factory.
Predictive Maintenance
AI-driven analysis can determine early signs of failure. This means that when an equipment is about to fail, manufacturers can replace it with a new one before any mishap.
Process Optimization
Digital twins also help manufacturers refurbish their operations and enhance efficiency. When a challenge occurs, the manufacturers work on strategies to handle it without worrying about anything else.
Enhanced Automation
Integrating digital twins and artificial intelligence allows innovative factories to adapt to various environments, ensuring a smooth workflow.
Key Components of Digital Twins
Manufacturing industries have noticed remarkable changes due to digital twins. This technology offers details about operations and physical assets. The best feature is that digital twins include components that help create a digital duplicate of physical entities.
Component | Description |
Physical Asset | A specific machine, manufacturing setting, or production line is duplicated. |
Digital Model | This digital representation of the physical asset provides insight into its functionality and structure. |
Data Connectivity | IoT sensors and data pipelines that ensure real-time data flow. |
AI & Machine Learning | Advanced algorithms analyze data for predictive maintenance, optimization, and decision-making. |
Simulation & Analytics | Computational models simulate various scenarios for predictive and prescriptive analytics. |
1. Physical Asset
What is the main attraction of a digital twin? It’s none other but the physical asset it represents. This asset could be a production line, an automated manufacturing setting, or a machine. The physical asset functions in real-time. This means it produces numerous data that consider its environmental conditions, operations, and performance.
For example, digital twins in a small factory can represent the following:
- Conveyor belts help transport materials from one place to another.
- CNC machines that manufacture suitable components.
- Industrial robots help monitor movements in an assembly line.
These physical assets are the primary data source gathered and entered into a digital model for regular examination.
2. Digital Model
The digital model is a virtual representation of the physical asset. It replicates the asset’s structure, behavior, and operational characteristics in a software-based environment. This model evolves in real time based on data collected from the asset, creating a dynamic rather than static representation.
A digital model includes:
- Geometric data (CAD models, 3D renderings).
- Behavioral data (how the asset functions under different conditions).
- Historical data (past performance, maintenance records).
For example, in an automotive manufacturing plant, a digital model of an engine assembly line would replicate the movement of robotic arms, the sequence of operations, and potential stress points in the process.
3. Data Connectivity
A crucial aspect of digital twins is seamless data connectivity between the physical asset and the digital model. This is made possible through:
- IoT Sensors: Devices embedded in machinery that capture temperature, vibration, pressure, energy consumption, and other key parameters.
- Data Pipelines: Secure and scalable infrastructure that transmits data from sensors to cloud or edge computing platforms in real time.
- Communication Protocols: Standards such as MQTT, OPC UA, and 5G that facilitate smooth data transfer.
Data connectivity ensures the digital twin is constantly updated with real-world conditions, enabling real-time monitoring and decision-making.
4. AI & Machine Learning
One of the most powerful elements of digital twins is using artificial intelligence (AI) and machine learning (ML) for predictive and prescriptive insights. AI-driven digital twins can:
- Detect patterns and anomalies in machine performance.
- Predict failures before they occur, enabling proactive maintenance.
- Optimize production schedules and resource allocation based on real-time demand.
For instance, in a steel manufacturing plant, AI models can analyze historical and real-time data to determine the best cooling rate for molten metal, ensuring optimal quality and minimizing defects.
5. Simulation & Analytics
A simulation engine within the digital twin allows manufacturers to test various scenarios without disrupting physical operations. It helps in:
- Scenario Planning: It simulates a production line’s behavior under different conditions, such as increased workload or supply chain disruptions.
- Process Optimization: Identifying bottlenecks and inefficiencies by analyzing production flow and material usage.
- Prescriptive Analytics: Providing recommendations on process adjustments to improve efficiency and reduce costs.
For example, a digital twin of a semiconductor fabrication plant can simulate how changes in environmental conditions (e.g., temperature or humidity) impact chip production yield. This allows engineers to adjust conditions proactively for better outcomes.
The Role of AI in Digital Twins
Integrating artificial intelligence into digital twins revolutionizes how manufacturers monitor, analyze, and optimize industrial processes. AI-powered digital twins go beyond static digital replicas by enabling automation, pattern recognition, predictive analytics, and real-time decision-making. These capabilities transform traditional factories into intelligent, self-learning ecosystems that improve efficiency, reduce downtime, and enhance overall operational performance.
Below, we explore how AI enhances digital twins in smart factories.
1. Predictive Maintenance: Avoiding Costly Downtime
One of AI’s most significant contributions to digital twins is predictive maintenance. In traditional manufacturing, maintenance is often performed on a fixed schedule or after equipment failure, leading to unplanned downtime and high repair costs. AI-driven digital twins change this by continuously analyzing machine sensor data and predicting failures before they happen.
How It Works:
- IoT sensors track machine health metrics such as temperature, vibration, and energy consumption.
- AI models process this data and detect early warning signs of wear, stress, or potential malfunctions.
- Maintenance teams receive alerts and recommendations for preventive actions before equipment breakdowns occur.
For example, in an automotive manufacturing plant, AI-driven digital twins can detect excessive motor vibrations in robotic arms and schedule repairs before a breakdown halts production.
2. Process Optimization: Enhancing Efficiency
AI enables digital twins to continuously optimize manufacturing processes by identifying inefficiencies, bottlenecks, and resource wastage. Traditional process optimization relies on human analysis, which can be slow and reactive. AI-driven digital twins, on the other hand, provide real-time recommendations for workflow enhancements and resource allocation.
How It Works:
- AI models analyze historical and real-time data from production lines.
- Machine learning algorithms detect patterns of inefficiency, such as excessive idle time or energy consumption.
- AI suggests optimizations such as adjusting machine speeds, reallocating tasks, or optimizing material flow.
For instance, in a food processing plant, AI-enhanced digital twins can adjust packaging line speeds based on real-time demand and inventory levels, reducing energy waste and increasing output efficiency.
3. Autonomous Decision-Making: Smart Manufacturing at Scale
AI-driven digital twins do not just assist human decision-makers—they can also act autonomously to optimize operations. By integrating AI, digital twins can self-regulate manufacturing parameters, adjust to changing conditions, and respond dynamically to production challenges.
How It Works:
- AI algorithms monitor production variables (e.g., temperature, pressure, material flow).
- When deviations occur, AI autonomously adjusts machine settings to maintain optimal performance.
- Smart contracts and AI models ensure quality and safety standards compliance without human intervention.
For example, AI-enabled digital twins can autonomously adjust temperature and chemical ratios during chip production in a semiconductor fabrication plant to maintain precision and minimize defects.
4. Anomaly Detection: Preventing Operational Disruptions
AI-powered machine learning models significantly enhance anomaly detection within digital twins. Instead of relying on pre-defined thresholds for detecting irregularities, AI automatically learns normal operating conditions and flags deviations.
How It Works:
- AI continuously analyzes real-time production data.
- Anomalies, such as unexpected machine vibrations or temperature spikes, are detected.
- Alerts are triggered, and AI provides possible causes and recommended corrective actions.
AI can be utilized in a steel manufacturing plant to identify microcracks in metal sheets that are invisible to the human eye. Additionally, the technology works like magic when maintaining top-notch product quality and lessening material waste.
5. Enhanced Simulations: Forecasting Performance & Outcomes
AI-driven digital twins have the skills to simulate several operational situations. These simulations offer noteworthy insights without hampering physical activities.
How It Works:
- Artificial intelligence models use past data to determine how several conditions hamper everyday tasks.
- Simulations are run to assess various scenarios, including sudden changes in raw material supply, machine breakdowns, and unexpected surges in demand.
- Manufacturers can identify the most efficient response by testing different options.
An automotive plant can use AI-powered simulations to determine how assembly line speed changes will influence employee productivity and output from manufacturing. This enables executives to make data-driven, informed choices.
Conclusion
AI-driven digital twins are redefining the future of manufacturing, enabling smart factories to achieve higher efficiency, predictive analytics, and autonomous decision-making. These virtual replicas are the key to reducing operational risks, optimizing production, and enhancing automation.
At Auxiliobits, we specialize in intelligent automation solutions, helping businesses leverage digital twin technology to transform operations with minimal disruptions. Are you ready to embrace the future of manufacturing? Contact us today to explore AI-driven solutions for your factory!