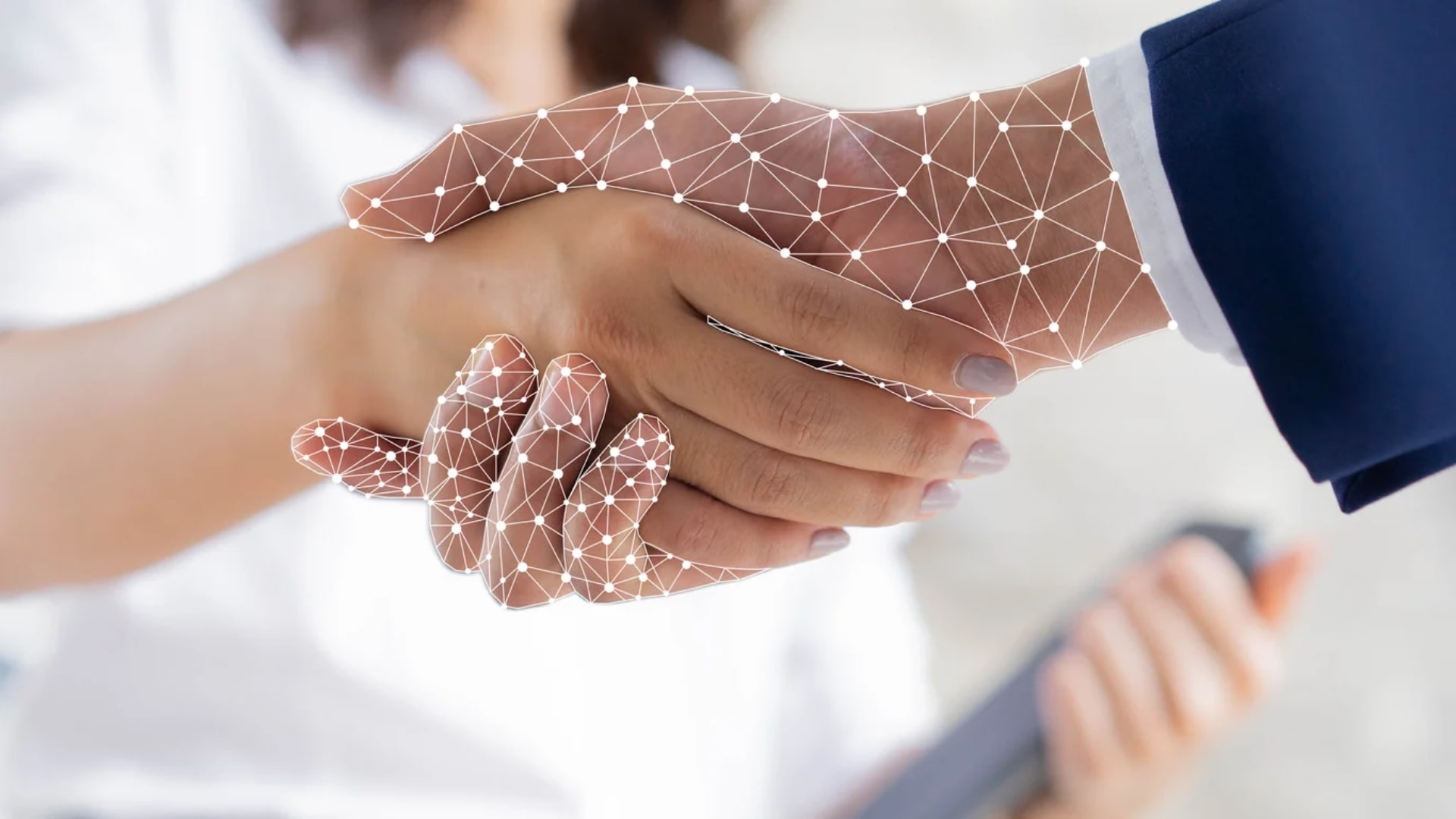
Key Takeaways
- AI-Augmented RPA empowers businesses to move beyond static automation by enabling systems that learn, adapt, and handle complex, non-linear processes with minimal manual intervention.
- Machine learning enhances automation resilience by enabling bots to make decisions rapidly, learn from historical data, and adapt to unpredictable scenarios—unlike rigid, rule-based RPA systems.
- ML-powered bots can handle unstructured data such as emails, scanned documents, and voice files using NLP and Computer Vision, expanding automation possibilities across departments.
- Continuous learning makes automation robust. It allows bots to adapt to changing interfaces and processes, reduces bot failures, and ensures consistent performance in dynamic business environments.
- AI-Augmented RPA improves scalability, accuracy, and speed, ensuring faster time to value, better ROI, and resilient operations even as data types, formats, and business needs evolve.
As companies try to keep up with digital changes, operational efficiency is one of everyone’s top priorities. One of the best technologies that is the foundation in this journey is robotic process automation (RPA). It has helped companies automate repetitive tasks and lessen manual intervention. Conventional RPA systems are effective in structured environments where procedures follow precise and consistent rules. Nevertheless, in today’s world, RPA solutions often reach their limitations and fail to meet businesses’ requirements. Business transformation relies heavily on AI-augmented RPA. It modifies the transformation procedure from reactive and static to adaptive and flexible. Additionally, it improves automation skills.
The results companies obtain are a new breed of automation with the skills and potential to handle non-linear processes and enhance intricate operations. AI-augmented RPA improves, learns, and identifies itself. This convergence allows companies to automate end-to-end processes while allowing the staff to focus on other tasks.
Also read: The Evolution of Intelligent Document Processing in Financial Services.
The Role of Machine Learning in Enhancing Resilience
As businesses aim for greater efficiency and agility through automation, integrating machine learning into robotic process automation has turned the tables upside down. It is machine learning that makes automation innovative, flexible, and brilliant. ML-augmented RPA systems have the potential to consider old data and learn from it as compared to conventional rule-based bots that depend on static logic. This is one primary reason this ability allows companies to deploy automation in previously complex situations for conventional RPA.
Let’s explore how ML helps in enhancing automation resilience. We have listed some of the key elements below:
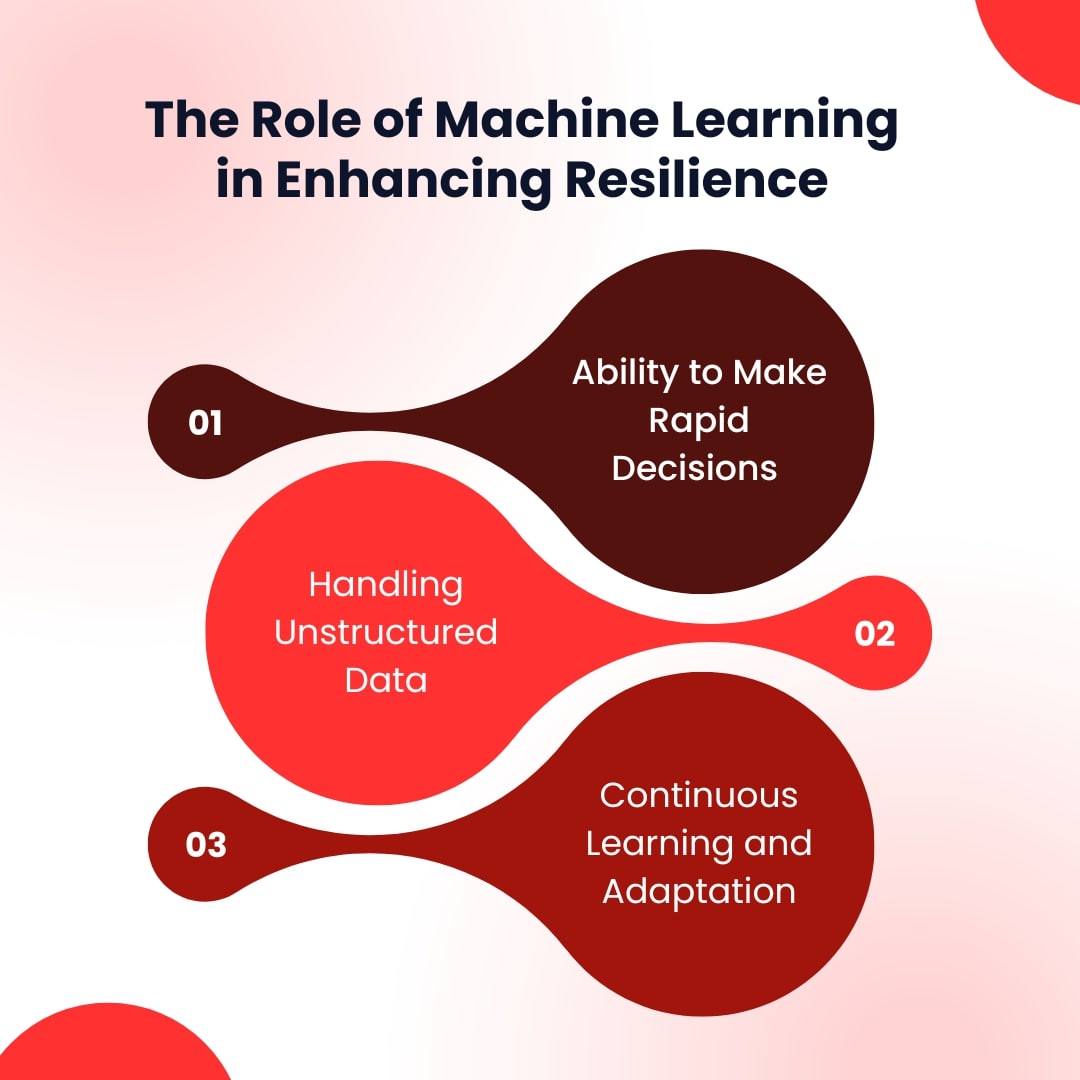
1. Ability to Make Rapid Decisions
One of machine learning’s best perks is its ability to make a final call without wasting time considering historical data. ML models are trained, allowing them to identify and respond to upcoming situations without explicit programming. By embedding these models into robotic process automation, firms help themselves make decisions in different environments.
For example, conventional RPA may encounter challenges with format variations. Additionally, it may face problems with incomplete fields when processing invoices. Nevertheless, ML-enhanced bots can recognize anomalies, including suspicious payments and duplicate invoices. This lessens human intervention and enhances operational accuracy.
2. Handling Unstructured Data
Over 80% of enterprise data is unstructured, residing in formats such as emails, scanned documents, images, and audio files. Rule-based bots often fail to process such data effectively because they lack contextual understanding. ML models, especially when combined with Natural Language Processing (NLP) and Computer Vision, enable bots to extract, classify, and interpret unstructured content with high precision.
For example, an AI-powered bot can read an email, understand the customer’s intent, categorize it into a relevant department, and generate an appropriate response. It can also process scanned receipts, handwritten notes, or even voice memos, expanding the scope of automation across various functions. This capability reduces errors, eliminates manual classification tasks, and increases end-to-end automation coverage.
3. Continuous Learning and Adaptation
One of traditional RPA’s significant drawbacks is its fragility—bots often break when user interfaces, workflows, or input formats change. ML addresses this limitation by enabling continuous learning. ML models can retrain themselves using fresh data, allowing bots to adapt to evolving business conditions without constant reprogramming.
This means that when a software interface updates or when user behavior shifts, ML-augmented bots can detect the change and adjust accordingly. This leads to robust automation pipelines that maintain high performance even in volatile environments, reducing downtime and maintenance costs.
Real-World Applications
Integrating machine learning into robotic process automation is transforming how enterprises approach automation. By enabling bots to learn from data, understand context, and adapt to changing environments, AI-Augmented RPA unlocks use cases across industries that were once considered too complex or variable to automate. Below are some key real-world applications where this powerful combination is driving measurable impact:
1. Customer Support Automation
AI-augmented bots can analyze the tone and sentiment of customer messages, prioritize urgent tickets, and route them to appropriate agents. This ensures consistent service quality and faster response times, even during high-volume periods.
2. Fraud Detection in Finance
ML-powered RPA bots can monitor thousands of transactions in real time, detect unusual behavior patterns, and trigger alerts or actions. This proactive approach enhances security and minimizes financial risks.
3. Healthcare Claims Processing
Bots equipped with ML can read and interpret medical records, validate claim forms, and detect inconsistencies. This accelerates claim settlement times while improving accuracy and compliance.
Benefits of AI-Augmented RPA for Business Resilience
In today’s digital economy, business resilience—the ability to adapt, recover, and thrive amid disruptions—is crucial. Traditional robotic process automation delivers value by automating rule-based, repetitive tasks. However, its limitations become evident in dynamic environments where processes shift, data is unstructured, and exceptions are the norm. AI-Augmented RPA addresses these gaps by combining the intelligence of machine learning and artificial intelligence with the efficiency of automation. This fusion brings powerful benefits that elevate automation effectiveness and enterprise resilience.
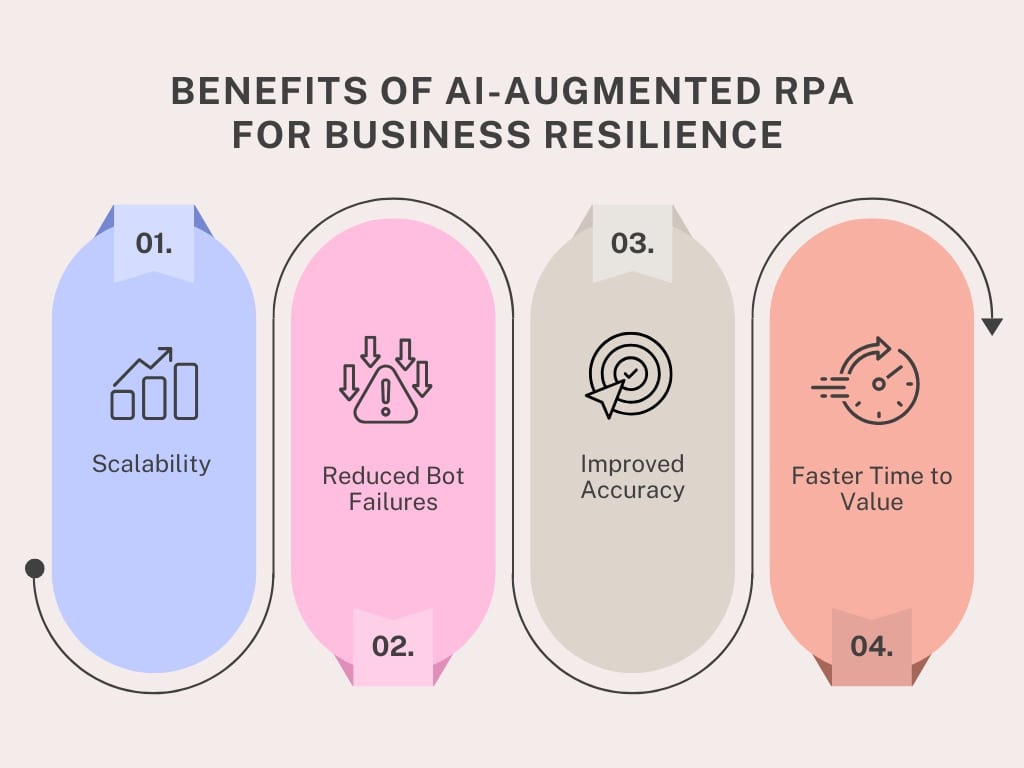
1. Scalability
AI-Augmented RPA enables organizations to scale their automation initiatives seamlessly. Traditional bots often struggle when they encounter variations in data types or volume increases. In contrast, ML-powered bots can manage a broad spectrum of structured and unstructured data, making automating more complex, data-intensive workflows possible.
For example, in customer onboarding or claims processing, where incoming documents vary in format and content, ML models can adapt and handle multiple formats without requiring additional coding. This flexibility supports rapid expansion of automation across departments, regions, and functions—without linear increases in development effort. As data volume grows, these bots continue to operate efficiently, ensuring that business operations remain smooth even under increased demand.
2. Reduced Bot Failures
One of the most critical advantages of ML integration is the reduction in automation breakdowns. Traditional RPA bots are brittle—they rely on fixed rules and screen layouts, making them vulnerable to minor UI or process logic changes. AI-augmented RPA changes this by embedding adaptability into the bot’s core behavior.
ML models detect and learn from anomalies, enabling bots to respond to changes in input data, interfaces, or workflows. Whether it’s a change in an invoice template or an updated form field on a website, ML-enhanced bots can adjust without requiring complete redevelopment. This reduces downtime, improves process continuity, and ensures business operations remain uninterrupted during transitions or disruptions.
3. Improved Accuracy
AI-Augmented RPA dramatically boosts the accuracy of automated processes. By learning from historical data, ML models can predict outcomes, identify patterns, and reduce the likelihood of human or rule-based errors. This results in more reliable outputs and fewer exceptions.
In finance or healthcare sectors, where precision is paramount, intelligent bots can validate data, flag inconsistencies, and ensure compliance with regulations. They don’t just follow rules—they make informed decisions based on real-time insights. This minimizes costly mistakes and improves overall process quality, directly contributing to customer satisfaction and operational trust.
4. Faster Time to Value
One key driver of digital transformation is speed—how quickly a business can go from idea to implementation. AI-augmented RPA accelerates this journey by reducing the need for manual configuration, testing, and exception handling. Bots can be deployed faster because they can understand context, make decisions, and learn from data without exhaustive programming.
Businesses can free up valuable resources and focus on innovation and strategic initiatives by minimizing human involvement in repetitive, data-heavy tasks. Moreover, intelligent bots can deliver measurable value from day one, ensuring a quicker return on investment (ROI) and enabling faster scaling of automation initiatives.
Also read: Evaluating Agentic AI in the Enterprise: Metrics, KPIs, and Benchmarks
The Ending Thoughts
AI-augmented RPA is a fundamental shift in how businesses approach automation. By infusing RPA with machine learning, enterprises gain systems that can learn, adapt, and thrive despite constant change. This integration empowers businesses with greater agility, resilience, and efficiency, helping them overcome the limitations of traditional automation and deliver sustained value across complex and dynamic environments.
The impact of ML-driven automation is profound, from handling unstructured data to reducing bot failures and ensuring faster time to value. As organizations face unpredictable market conditions and rising customer expectations, building resilient operations through AI-augmented RPA becomes a competitive advantage and a necessity.
Are you ready to enhance your automation strategy? Look no further and get in touch with Auxiliobits today.