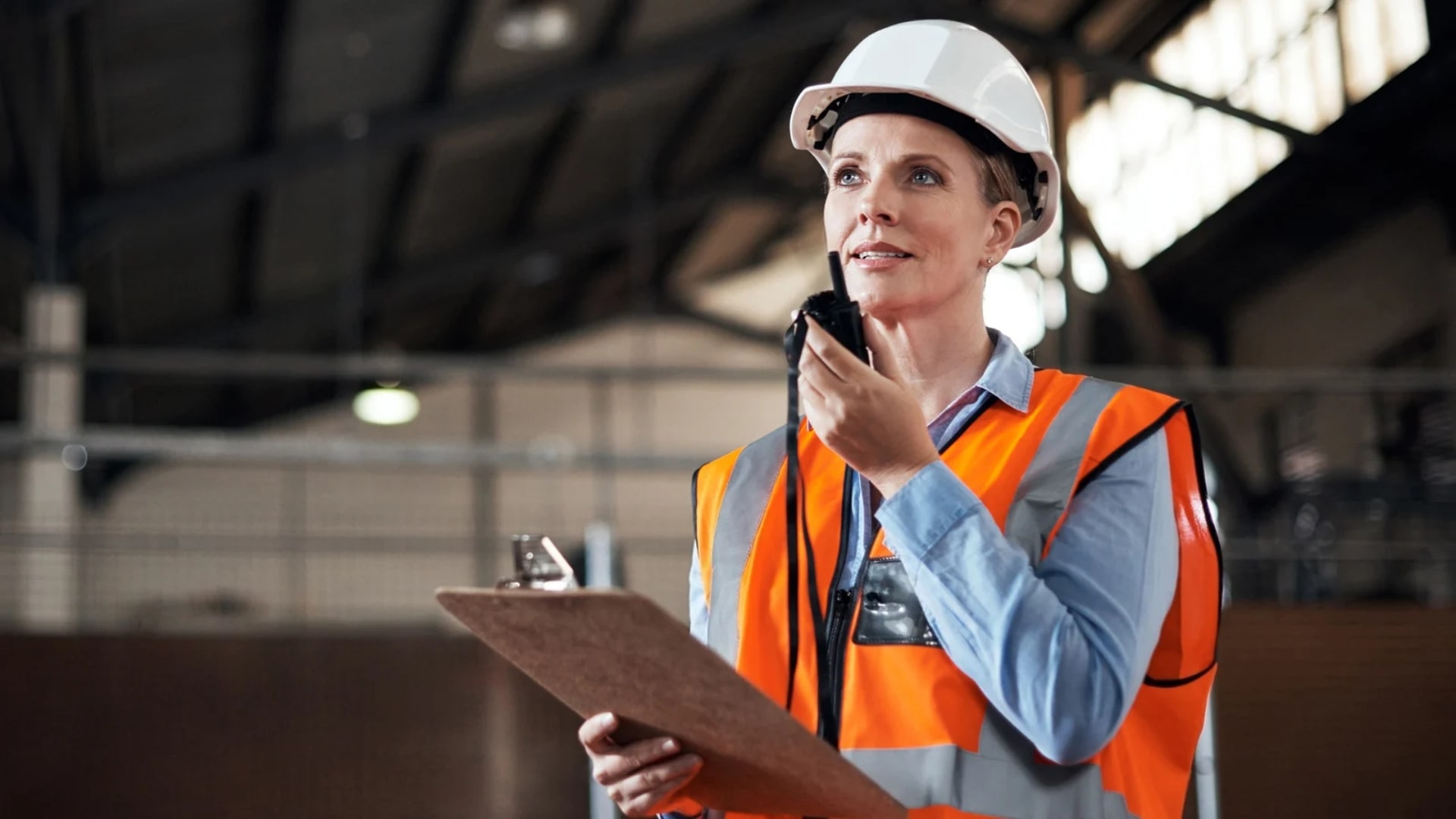
Key Takeaways
- Multi-agent AI enables real-time, data-driven decisions to optimize inventory, logistics, and procurement, reducing disruptions and improving overall supply chain efficiency.
- By distributing decision-making, AI systems enhance resilience, ensuring supply chains remain operational even during failures, supplier issues, or unexpected disruptions.
- AI-driven analytics forecast demand shifts, detect risks like weather disruptions, and proactively adjust supply chain strategies to prevent bottlenecks and inefficiencies.
- AI-powered inventory and logistics management minimizes waste, lowers storage and fuel costs, and improves sustainability through adaptive process automation and intelligent routing.
- AI automates communication, ensuring suppliers, manufacturers, and logistics partners stay synchronized, reducing delays and improving procurement and production workflows.
Did you know that multi-agent artificial intelligence systems (MAS) are redefining supply chain optimization for the better? If we consider the global market, supply chains are the most intricate. Therefore, MAS is handy here because it addresses inefficiencies and vulnerabilities in traditional supply chain management. These systems also help avoid several challenges because conventional tools face severe challenges when adapting to changing conditions. One primary reason behind this is the dependence of traditional tools on past information. Multi-agent intelligence systems work wonders by functioning independently with the help of machine learning algorithms.
Firms integrating multi-agent AI into supply chain management can enjoy benefits like resilience, adaptability, and improved efficiency. Additionally, firms will learn to explore uncertainties, enhance workflow, and set different goals.
Also read: Evaluating Agentic AI in the Enterprise: Metrics, KPIs, and Benchmarks
Key Characteristics of Multi-Agent AI Systems
Now that you know what multi-agent AI systems are, let us shed some light on their key characteristics. We have listed them below:
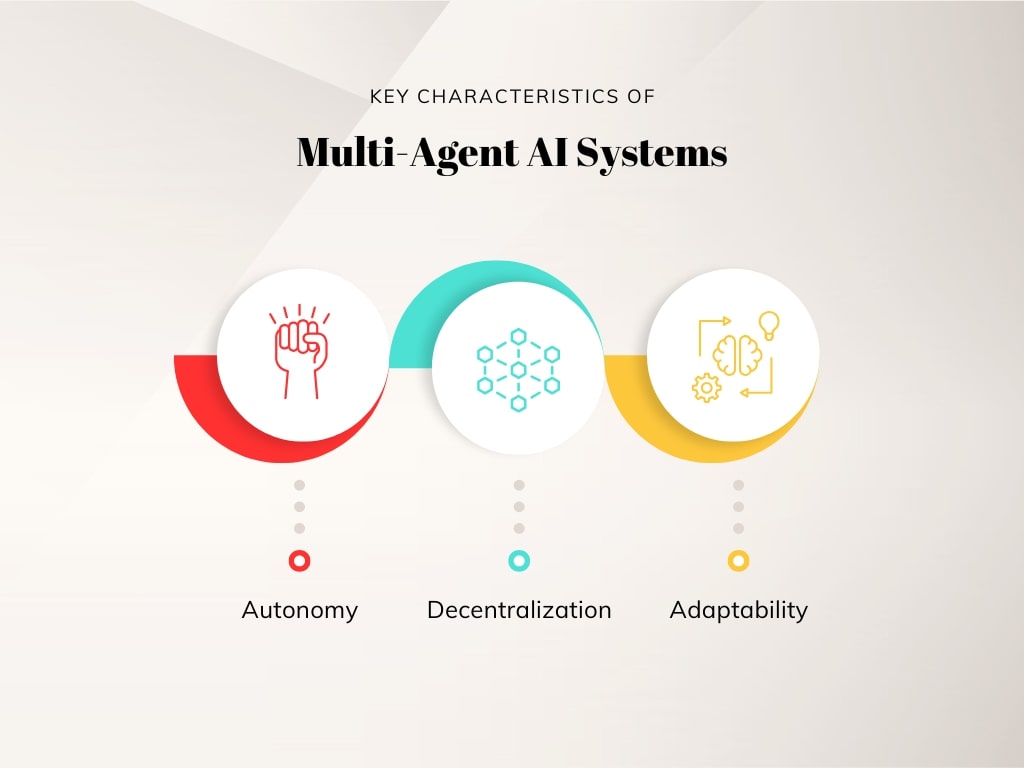
1. Autonomy
MAS functions individually. This means that these systems do not ask for any human intervention. Every single agent has the requirements with itself. They not only have predefined objectives but also predict demand changes. Additionally, these agents have the expertise to make decisions using ERP systems, IoT sensors, and market changes.
For example, logistics professionals can consider weather problems and traffic situations to ensure that all shipments are completed on schedule.
2. Decentralization
Conventional supply chains depend on centralized control, which increases the chances of failure and inefficiency. MAS, nevertheless, distributes decision-making among several agents who work independently. This improves system resilience and ensures a smooth workflow, even when things don’t fall into place.
For example, if a provider faces challenges and production delays, AI agents can recognize this and look for other suppliers. This lessens vulnerabilities, and the supply chain robustness also improves.
3. Adaptability
Supply chains operate in highly dynamic environments influenced by market trends, geopolitical shifts, and natural disasters. Multi-agent AI systems exhibit adaptability by learning from historical and real-time data, enabling them to adjust strategies as needed.
Using reinforcement learning techniques, these agents continuously refine their decision-making models to improve performance. For example, a demand-forecasting agent can adapt its predictions based on evolving consumer behavior, ensuring optimal inventory management and reducing waste.
Benefits of Multi-Agent AI Systems in Supply Chain Management
In today’s unpredictable global market, supply chains require agility, intelligence, and automation to remain competitive. Traditional supply chain management systems often struggle with inefficiencies, disruptions, and communication gaps.
Multi-agent AI Systems (MAS) provide a game-changing approach by enabling autonomous decision-making, predictive analytics, and seamless collaboration across the supply chain. These intelligent systems enhance operational efficiency, reduce costs, and improve supply chain resilience. Below are the key benefits of MAS in supply chain management.
1. Enhanced Decision-Making
One of MAS’s primary advantages is its ability to make real-time, autonomous decisions based on data-driven insights. These AI agents analyze vast amounts of data from multiple sources—IoT sensors, market trends, and logistics networks—to optimize supply chain operations.
For example, if a sudden surge in demand occurs, AI agents can automatically adjust procurement and production schedules to prevent stockouts. Likewise, in a disruption—such as a supplier delay or geopolitical conflict—these systems can swiftly identify alternative suppliers and reroute shipments, ensuring business continuity. This real-time adaptability enables companies to respond more efficiently and precisely to market fluctuations.
2. Optimized Inventory Management
Maintaining optimal inventory levels is crucial to avoid excessive storage costs and lost sales opportunities. MAS leverages predictive analytics to forecast demand accurately and adjust inventory levels accordingly.
AI-driven inventory management prevents overstocking and understocking by analyzing historical sales data, customer buying patterns, and external factors like seasonal demand shifts. For instance, a major retailer using MAS successfully reduced stockouts by 30% during peak shopping seasons by redistributing inventory in real time based on regional demand fluctuations. This optimization level ensures that products are available where they are needed most, improving customer satisfaction and reducing waste.
3. Improved Supplier Collaboration
Supply chains involve multiple stakeholders, including suppliers, manufacturers, distributors, and retailers. Effective communication and coordination among these entities are essential for smooth operations. MAS facilitates seamless supplier collaboration by enabling automated communication and data exchange.
AI agents can instantly share production schedules, delivery timelines, and demand forecasts with suppliers, ensuring that supply chain partners are aligned. For example, AI agents can automatically notify suppliers and arrange expedited deliveries if a manufacturing facility requires additional raw materials due to increased production demand. This reduces delays, enhances efficiency, and strengthens supplier relationships.
4. Predictive Risk Mitigation
Disruptions such as supply shortages, transportation delays, and natural disasters can severely impact supply chain operations. MAS proactively analyzes potential risks by continuously monitoring real-time data from various sources, including weather forecasts, geopolitical developments, and economic trends.
By implementing machine learning and predictive analytics, these systems can anticipate disruptions and implement proactive strategies to minimize impact. For example, an AI-driven logistics system can detect potential delays caused by adverse weather conditions and automatically reroute shipments to prevent delivery bottlenecks. This predictive approach ensures supply chain continuity and minimizes financial losses
5. Cost Efficiency
Implementing MAS leads to significant cost savings by optimizing supply chain processes, reducing waste, and improving resource utilization. AI-driven route optimization helps reduce fuel costs by identifying the most efficient delivery routes, while intelligent inventory management minimizes excess stock and storage expenses.
A logistics company using MAS reduced fuel costs by over $500,000 annually by dynamically rerouting shipments based on real-time traffic and weather conditions. Similarly, manufacturers can reduce labor and operational costs by automating routine supply chain tasks, such as order processing, scheduling, and demand forecasting. By eliminating inefficiencies and enhancing automation, MAS drives overall cost reduction while improving supply chain performance.
Also read: The Role of Large Language Models (LLMs) in Agentic Process Automation
Applications in Supply Chain Optimization
The modern supply chain is an intricate network of suppliers, manufacturers, logistics providers, and retailers that must function seamlessly to meet market demands. However, traditional supply chain management approaches often struggle with inefficiencies, unforeseen disruptions, and sustainability challenges. Multi-agent AI systems transform supply chain operations by enabling autonomous decision-making, predictive analytics, and real-time optimization. These intelligent systems enhance efficiency, resilience, and sustainability across the supply chain ecosystem. Below are five key applications of agentic AI in supply chain optimization.
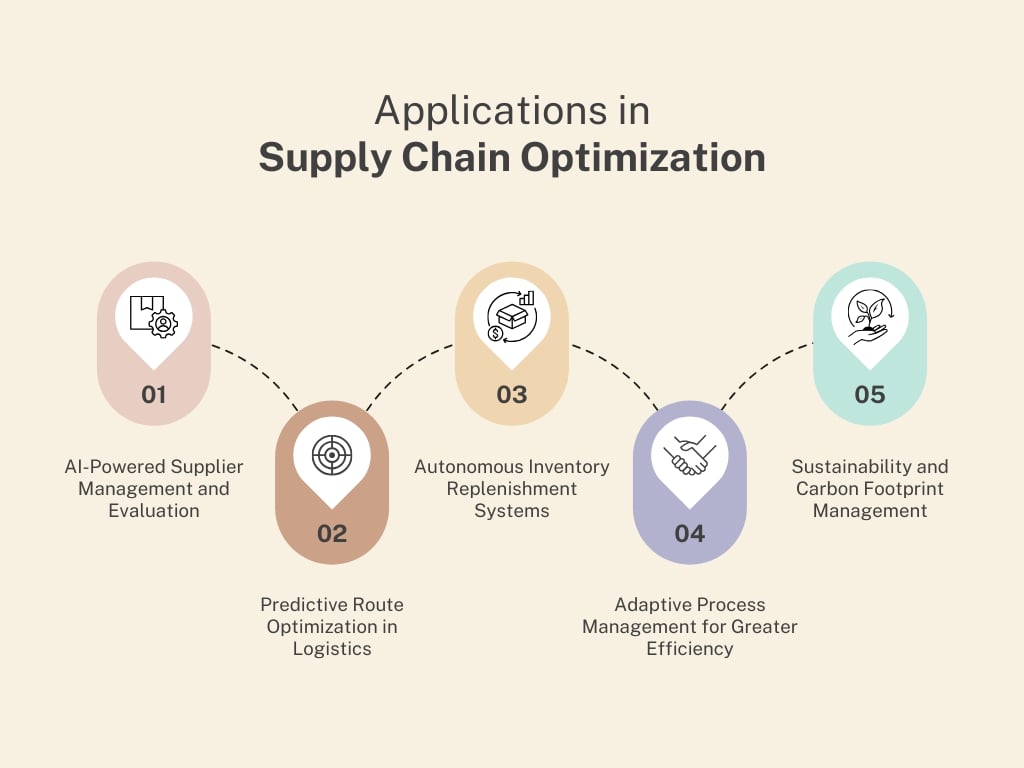
1. AI-Powered Supplier Management and Evaluation
Selecting and managing suppliers is critical to supply chain management, influencing procurement efficiency, product quality, and overall operational performance. Agentic AI systems autonomously assess supplier performance by analyzing key metrics such as delivery punctuality, quality compliance, and sustainability adherence.
For instance, an automotive company implemented an AI-driven supplier evaluation system that continuously monitored supplier reliability and quality performance. The company improved procurement efficiency by 20% by leveraging AI-driven insights, ensuring that only the most reliable suppliers were onboarded. This streamlined the procurement process and reduced supply chain risks by proactively identifying underperforming suppliers.
2. Predictive Route Optimization in Logistics
Logistics disruptions due to transportation delays, adverse weather, and geopolitical events can have significant cost implications. Multi-agent AI systems mitigate these challenges by continuously analyzing real-time traffic conditions, weather patterns, and regulatory changes to optimize delivery routes.
A logistics provider implemented an AI-powered multi-agent system to reroute shipments based on live traffic and weather data. During a severe weather event, the AI system automatically adjusted delivery schedules and rerouted shipments, reducing delays by 25% and generating annual fuel savings of over $500,000. AI significantly enhances delivery efficiency by optimizing transportation logistics while minimizing operational costs.
3. Autonomous Inventory Replenishment Systems
Maintaining the proper inventory levels is essential to prevent stockouts and overstocking, which lead to revenue loss and increased storage costs. Agentic AI systems analyze sales patterns, market trends, and real-time demand fluctuations to optimize inventory management.
A global retailer adopted an AI-powered inventory replenishment system during the holiday season. The system detected regional demand surges and automatically redistributed products across different store locations. This intelligent redistribution reduced stockouts by 30%, ensuring better customer satisfaction while minimizing inventory waste. AI-driven inventory management enables businesses to proactively respond to demand shifts, improving overall supply chain agility.
4. Adaptive Process Management for Greater Efficiency
Supply chains involve complex, interconnected processes across manufacturing, warehousing, and distribution networks. Agentic AI optimizes process management by decentralizing decision-making, allowing local facilities to adjust production schedules and resource allocation autonomously.
For example, a consumer electronics brand deployed an AI-driven system to optimize production schedules across multiple factories. By leveraging real-time demand data and operational analytics, the system improved production efficiency by 15% and reduced downtime, ensuring optimal resource utilization. AI-driven process management minimizes bottlenecks and enhances overall supply chain efficiency.
5. Sustainability and Carbon Footprint Management
Sustainability is an increasingly important focus for supply chain operations, with companies aiming to reduce carbon emissions and optimize energy consumption. Multi-agent AI systems help organizations achieve these goals by selecting eco-friendly transportation routes, optimizing fuel usage, and monitoring energy efficiency.
A global beverage company implemented AI-powered sustainability monitoring to track energy consumption across its supply chain. By analyzing transportation emissions and production energy usage, the AI system identified areas for optimization, leading to a 12% reduction in CO2 emissions and a 10% improvement in delivery efficiency. AI-driven sustainability initiatives enhance environmental responsibility and contribute to long-term cost savings and regulatory compliance.
The Last Words
Companies can optimize logistics, inventory, procurement, and supplier collaboration using autonomous AI agents while proactively mitigating risks. At Auxiliobits, we specialize in AI-driven supply chain transformation, using agentic process automation (APA) and intelligent automation to help businesses enhance their supply chain efficiency. Contact us to learn more.