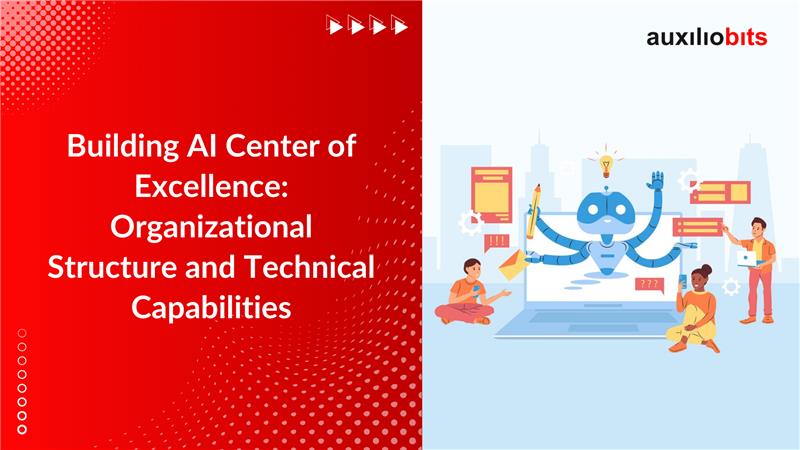
What if your company could make the best possible use of artificial intelligence through a dedicated hub of skills, expertise, innovation, and teamwork? An AI Center of Excellence (CoE) can achieve this by acting as a central hub that combines resources, best practices, and knowledge for AI initiatives. The CoE helps enterprises in growing their AI initiatives. In addition to this, they also ensure compliance and a strong return on investment. Creating an AI CoE necessitates a blend of domain knowledge, data science skills, and technical resources. Leadership roles within the organizational structure typically include AI strategists, data scientists, machine learning engineers, and governance experts. These specialists work together to deliver appropriate solutions that satisfy client and organizational objectives.
On the other hand, an AI CoE is also responsible for developing and upholding valid infrastructure for training and deployment purposes. This architecture makes strong data pipelines and incorporates machine learning frameworks. The CoE ensures that artificial intelligence practices are used wisely and monitors them using suitable platforms and tools. No matter where your company is on its AI journey, a well-organized AI Center of Excellence (CoE) can be a game-changer.
Also read: Why Is UiPath Integration with Chatbots and Virtual Assistants Non-Negotiable?
Key Objectives of an AI CoE
An AI Center of Excellence is a vital initiative for companies seeking to transform with artificial intelligence. Some of the primary objectives of an AI CoE are mentioned below. Check them out:
1. Innovation Acceleration
The foremost objective of an AI CoE is to accelerate innovation by creating and deploying AI solutions that address complex company challenges. Some enterprises are unable to make use of the opportunities they deserve. This is where the CoE comes in handy by enabling collaboration among engineers, data scientists, and business stakeholders. This collaboration can help companies experiment with the latest technologies and how they can benefit them. That said, let us now explore the crucial aspects of innovation acceleration. Below mentioned are some of them:
- Recognizing and monitoring use cases in various departments to gain access to better decisions, predictive analytics, and automation.
- Contact different technology partners and research schools to implement current AI advancements.
- Piloting artificial intelligence models to validate business impact.
2. Standardization
Standardization is paramount because it ensures compliance in AI deployment and development. Apart from this, it also ensures quality and consistency. The CoE creates business-wise standards for artificial intelligence models, governance, and data management. Some of the key standardization responsibilities consist of the following:
- Explaining KPIs so that the success of artificial intelligence can be examined.
- Adopting model operations to keep a regular check on lifecycle management.
- Establishing AI assets that have already been used, including APIs, data pipelines, and pre-built models.
- Preparing AI governance frameworks that include compliance regulations, transparency measures, and ethical guidelines.
3. Talent Development
When implementing artificial intelligence correctly, businesses need specialized AI ethics, data engineering, and machine learning. One of the foremost objectives of an AI CoE is to build and nurture AI talent within all types of firms. This includes the below mentioned:
- Providing everything from workshops, training programs, and achievement certificates in artificial intelligence and tools.
- Promoting AI literacy.
- Creating AI communities for learning.
- Offering mentorship opportunities.
- Promoting cross-functional collaboration.
4. Strategic Alignment
AI CoE is also responsible for ensuring that artificial intelligence initiatives align with company goals. AI projects do not have value if there is no suitable alignment. In addition, companies are wasting their time because their projects will be at stake. The CoE bridges the gap here by collaborating with business units. Some of the strategic alignment efforts that are made consist of the following:
- Considering long-term objectives.
- Aligning AI investments with company goals.
- Not taking AI use cases for granted.
- Ensuring transparency between companies and stakeholders.
- Refining AI strategies when needed.
- Adapting AI strategies to market changes.
5. Risk Management
With AI deployment comes various risks, operational problems, legal issues, etc, to name a few. One of the objectives of a CoE is to mitigate these risks by implementing applicable AI practices. In addition, a CoE will also manage everything to ensure that the company’s operations are not hindered. Key risk management activities consist of the following:
- Conducting mitigation.
- Performing bias detecting.
- Establishing security protocols.
- Monitoring AI systems for anomalies.
- Implementing AI ethics rules and regulations.
Also read: Enhancing Enterprise Agility with RPA Automation in AI-driven Business Architectures
Organizational Structure of an AI CoE
The structure of an AI CoE typically consists of four key components. They are mentioned below:
1. Governance Model
A strong governance model ensures alignment between AI projects and organizational goals. It involves defining policies, standards, and AI development and deployment guidelines. Explore the table below to learn more:
Governance Compliance | Explanation |
Steering Committee | Includes senior leaders providing oversight. |
AI Ethics and Compliance Board | Ensures better use of artificial intelligence and regulatory compliance. |
Project Management Office | Manages resources, budgets, and AI project timelines. |
2. Core Team Roles
Role | Responsibilities |
AI CoE Lead | Responsible for developing strategies and adaptation. |
Data Scientists | Accountable for developing AI models and performing advanced analytics. |
ML Engineers | Build and deploy AI models into production. |
Data Engineers | Ensure data quality and manage data pipelines. |
Business Analysts | Bridge the gap between technical teams and business stakeholders. |
AI Ethicists | Make sure that AI apps adhere to ethical regulations. |
3. Cross-Functional Collaboration
An AI CoE must collaborate with various IT, operations, marketing, finance, and legal departments. This cross-functional collaboration ensures AI solutions are integrated seamlessly across the enterprise.
Technical Capabilities for AI CoE
Building a robust AI CoE requires investing in the right technical capabilities, including infrastructure, tools, and platforms.
1. Infrastructure and Tools
- Cloud Platforms: AWS, Azure, or Google Cloud for scalable AI model training and deployment.
- AI Frameworks: TensorFlow, PyTorch, and Scikit-learn for model development.
- MLOps Tools: Kubeflow, MLflow, or Databricks for end-to-end model management.
2. Data Management
- Data Lakes and Warehouses: Store large datasets for AI model training.
- Data Governance Tools: Ensure data quality, security, and compliance.
- ETL Pipelines: Extract, transform, and load data for AI consumption.
3. AI Model Development
- Implement robust model lifecycle management.
- Use AutoML to accelerate model creation.
- Leverage Explainable AI (XAI) for model transparency.
4. Model Monitoring and Maintenance
- Setup monitoring systems to detect model drift and performance issues.
- Implement continuous learning to update models with new data.
Best Practices for Establishing an AI CoE
Establishing an AI Center of Excellence (CoE) is a strategic move for organizations seeking to harness the power of artificial intelligence for innovation and growth. An AI CoE is a centralized unit that provides leadership, governance, and support for AI initiatives across the enterprise. Here are the best practices for setting up a successful AI CoE.
1. Define Clear Objectives and Vision
- Establish a well-defined vision and objectives aligned with the organization’s strategic goals.
- Focus on how AI can solve business challenges, improve processes, and create value.
- Set measurable KPIs to track the CoE’s success.
2. Secure Executive Sponsorship
- Gain support from senior executives to ensure alignment with business priorities.
- Executive sponsors can provide funding, remove roadblocks, and promote AI adoption across departments.
3. Form a Multidisciplinary Team
- Build a diverse team with AI specialists, data scientists, engineers, business analysts, and domain experts.
- Encourage cross-functional collaboration to address various AI use cases.
- Invest in continuous training to upskill employees in AI technologies.
4. Establish Strong Governance
- Develop clear governance policies for AI development, deployment, and monitoring.
- Implement ethical guidelines and ensure compliance with regulatory requirements.
- Define roles and responsibilities to ensure accountability and transparency.
5. Create a Scalable Infrastructure
- Invest in scalable AI infrastructure, including cloud platforms, data lakes, and machine learning tools.
- Ensure seamless integration with existing enterprise systems.
- Leverage MLOps for efficient model deployment and management.
6. Foster Innovation and Experimentation
- Encourage a culture of experimentation by running AI pilot projects and proof-of-concepts (PoCs).
- Establish an AI sandbox environment for testing new ideas without impacting production systems.
- Learn from failures and iterate quickly to improve AI solutions.
7. Ensure Data Accessibility and Quality
- Implement robust data management practices to ensure data quality, availability, and security.
- Break down data silos to provide AI teams with comprehensive datasets.
- Use AI-powered data governance tools to automate data classification and compliance.
8. Standardize AI Development
- Develop reusable AI models, frameworks, and components to accelerate development.
- Establish standardized coding practices and model documentation.
- Promote the use of AI platforms and APIs to ensure consistency.
9. Monitor and Optimize AI Performance
- Implement monitoring tools to track AI model performance in real-time.
- Continuously retrain models using fresh data to maintain accuracy.
- Use AI explainability tools to ensure transparency and build trust.
10. Drive Enterprise-Wide Adoption
- Conduct workshops and training programs to build AI literacy across the organization.
- Develop a knowledge-sharing portal to disseminate AI best practices and success stories.
- Collaborate with business units to identify and prioritize AI use cases.
The Ending
Are you ready to adapt for your enterprise? Partner with Auxiliobits to build a future-ready AI CoE that enables innovation ensures compliance, and delivers measurable business outcomes.