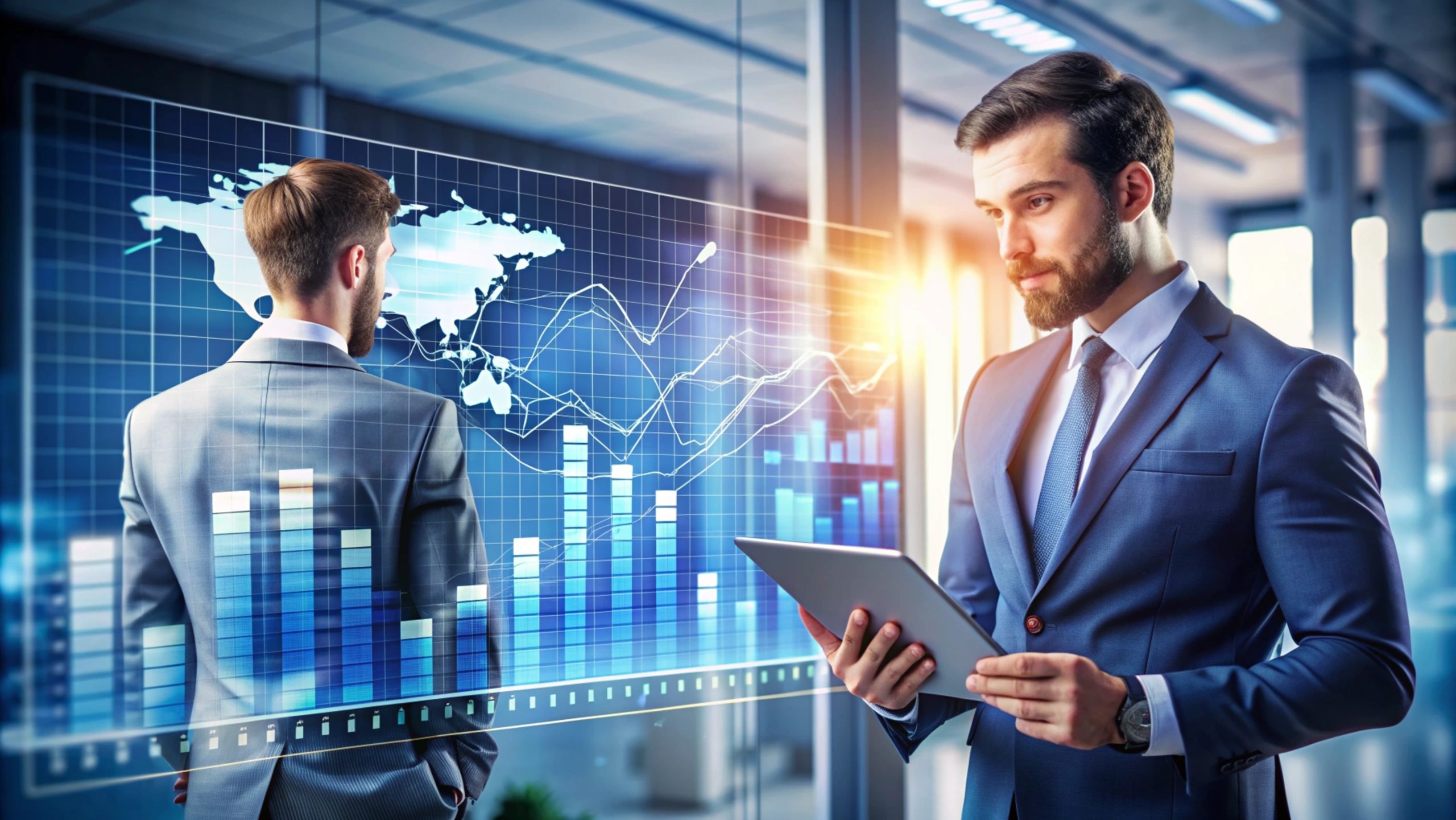
As artificial intelligence (AI) technology advance, two concepts regularly appear in discussions: generative AI and large language models (LLMs). They are closely linked and often stated together, but they are not the same thing. Understanding the difference between Generative AI and LLMs is critical for comprehending how current AI systems work and their many uses. In this article, we’ll break down these notions, look at their distinguishing traits, and explain how they interact.
What is generative AI?
Generative AI, an innovative branch of AI, refers to any AI system that can generate new content using the data it has learnt from. These models provide unique outputs in the form of text, graphics, audio, video, or code. Unlike standard AI models, which concentrate on classification or regression (e.g., recognising an item or predicting a value), generative AI generates something new rather than just identifying or analysing existing data.
For example, a generative AI model for may create a realistic picture of a cat that does not exist in real life based on its knowledge of what a “cat” looks like after analysing millions of photographs.
Applications for Generative AI:
Text creation entails creating articles, tales, or news reports.
- Image creation includes making art, producing commercials, and generating deepfake videos.
- Music composing include creating unique songs or background soundtracks.
- Video creation includes animation, video editing, and even full-length films.
- DALL·E is a well-known generative AI model that uses textual descriptions to create unique and detailed visuals.
What is a Large Language Model (LLM)?
Large Language Models (LLMs) are a kind of generative AI model that is especially designed to interpret and generate human language offering ai automation services. LLMs are trained on large datasets of text from books, webpages, and other written languages, allowing them to recognise complicated language patterns, syntax, and even some amount of contextual meaning. Their potential to revolutionize language-related tasks is immense.
Language learning machines process language using advanced approaches such as deep learning. A typical LLM can answer questions, translate languages, summarise material, and even have human-like conversations. OpenAI produced LLMs like as GPT-3 (Generative Pre-trained Transformer 3) and GPT-4, which are widely used for tasks such as language translation, content summarization, and question answering.
Key differences between generative AI and LLMs – AI vs. Large Language Models
While generative AI and LLMs both generate material, their scope, functionality, and applications vary significantly.
1. Scope: Generative AI refers to any AI system that can generate new data in several domains, including text, pictures, audio, and video.
LLMs is particularly design to generate and comprehend human language.
2. Output: Generative AI may generate pictures, audio, music, video, and 3D objects, in addition to text.
LLMs specialise in creating text-based outputs such conversations, reports, and translations.
3. Underlying Architecture: LLMs and other generative AI models use neural networks, namely deep learning models. However, LLMs such as GPT-4 use Transformer designs, which are especially well-suited to processing sequential data (such as language) and big datasets.
Generative AI models for pictures, such as GANs (Generative Adversarial Networks) and VQ-VAE (Vector Quantised Variational AutoEncoders), use architectures that are more suited to processing pixel data.
4. Training Data: Generative AI models may trained on numerous data formats, such as photos, audio, or video, based on the desired output.
LLMs are trained on vast text data sets and fine-tuned for tasks like as question answering, essay authoring, and content summarisation.
How Do LLMs Fit Into Generative AI?
LLMs form a subset of generative AI. They are one of the numerous kinds of AI models capable of creating new material, but their expertise is in language. In principle, every LLM is a generative AI model; yet, not all generative AI models are LLMs.
Consider this: an AI that can produce a photorealistic picture of a cityscape from a basic description (e.g., “a sunset over a city with tall buildings”) is a generative AI model for photos. In this way it offers robotic process automation services. On the other hand, an AI that develops a creative short tale from the same description is most likely an LLM—both models generate content, but the sort of material and underlying technology vary.
Real-World Applications: Generative AI vs. Large Language Models
Understanding the distinction between these two AI of business automation services categories may aid in identifying their specific applications in various sectors.
Generative AI Applications:
Art and design professionals employ generative AI to generate original digital art, animations, and logos.
Gaming uses generative AI to create virtual environments, non-player characters (NPCs), and in-game assets such as landscapes and goods.
Content Creation: Generative AI is usd by social media platforms and marketers to automatically create pictures and videos targeted to their intended audience.
Product Design: Companies such as Nike employ generative AI to produce new shoe designs based on consumer preferences and past product trends.
LLM Applications:
Customer Support: LLMs ai for customer service enable chatbots that may respond to customer enquiries, walk users through troubleshooting processes, and make suggestions.
Journalists and content makers utilise LLMs to swiftly compose news pieces, blog entries, and reports, as well as for research purposes.
Language models facilitate the accurate translation of text from one language to another.
Education: LLMs may give explanations and tutoring in a variety of areas, allowing students to learn complicated issues in an interactive setting.
The Future of Generative AI vs. Large Language Models
The future of AI will most likely entail increased integration of generative AI with LLMs. As the models advance, their applications will expand, from personalised education and creative media to healthcare diagnostics and scientific research. This integration could lead to more sophisticated AI systems that can understand and generate content in multiple modalities, revolutionizing industries such as entertainment, education, and healthcare.
Multi-modal models are already becoming more popular, with models capable of interpreting and producing text, graphics, and audio all at the same time. ChatGPT, which is based on LLM but also includes picture production and audio elements, is one example of this progression. Such developments further blur the barrier between generative AI and LLMs, making it more complicated.
Conclusion
Generative AI and LLMs both concentrate on content production, although in distinct methods and circumstances. To sum up, generative AI is a broad category that includes any AI system capable of generating new content, while LLMs are a specific type of generative AI that is design to understand and produce human language. If you want to leverage both, Auxiliobits offers business automation services leveraging ai.
We are here to drive advances in sectors ranging from entertainment and education to healthcare and beyond.